False positives are the bane of most fraud management team's existence — 27% of risk professionals said it’s their biggest pain point related to fraud. Risk and compliance professionals are constantly fighting to keep false positives rates low and under control, while still making sure they aren’t letting fraud pass through undetected.
Frankly, false positives can have a massive impact on an organization, leading to lost revenue, reputational damage, and wasted resources and expenses. We’ll explore the foundational information about false positives in fraud and AML so you can work towards mitigating false positives.
To start, let’s look at what false positives are in the context of fraud and AML compliance.
What is a False Positive in Fraud?
A false positive in fraud occurs when a legitimate transaction is incorrectly classified as potentially fraudulent. In the most basic terms, a false positive indicates a suspected incident of fraud, when in fact, no fraud has actually occurred.
False positives — in the context of fraud — represent lost sales, lost revenue, and misplaced fraud prevention efforts. Risk professionals also use false positives to gauge how effective their anti-money laundering efforts are, especially when using tools like transaction monitoring.
Although it’s crucial to treat every fraud alert seriously and investigate each case, false positives can add a considerable burden on risk management teams. Without enough staff to sufficiently manage alerts, you’ll quickly find yourself overwhelmed by alert backlogs — and once they start piling up, they’re hard to stop. Teams failing to manage their alerts effectively could find themselves struggling to meet regulatory requirements, efficiently delegate resources, and deliver adequate fraud protection.
The more false positives you’re investigating, the less your resources are being devoted to real fraudulent activity. With the need to create, test, and implement new rules on a constant basis, false positives can create a considerable workload for risk management teams.
And the worst part is that these efforts aren’t actually stopping fraud — they’re stopping legitimate customers.
How to Calculate False Positives
The false positive rate is extremely useful for risk and compliance teams. It empowers teams to determine how successful their fraud and AML programs are, as well as pinpoint their weaknesses. With this information, risk management teams can optimize improvements and reduce fraud false positives.
The false positive rate is calculated using the following formula:
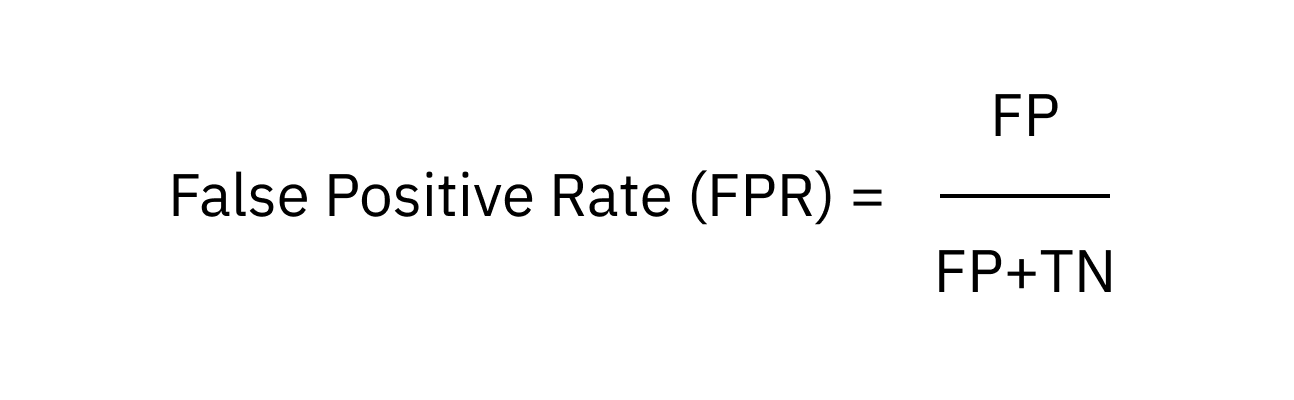
Where:
- FP is the number of False Positives in a given time period
- TN is the number of True Negatives in a given time period
It’s also helpful to think of the total number of false positives and true negatives (FP + TN) as its own value — the total number of negative results (N).
Let’s look at an example to illustrate. A company experiences 200 false positives (FP) and 800 true negatives (TN) in a month. To calculate their false positive rate, they need to divide the number of false positives by the total number of negatives (N), which is the sum of the 200 false positives (FP) and the 800 true negatives (TN). Therefore, the total number of negatives (FP + TN) is 1,000. Next, we need to divide the number of false positives (FP) by the total number of negatives (N). This would be 200 false positives divided by 1,000 negatives, which would give us a false positive rate of 20%.
It’s important to keep in mind that when performing this calculation, you have to be looking at a specific time period. Whether that’s a week, a month, or a year, the important thing is that all values are analyzed in the same time frame.
By calculating the false positive rate, you can assess the efficiency of the fraud detection systems and make necessary adjustments to minimize false positives. A high false positive rate means a large number of legitimate transactions are being flagged as fraudulent, leading to dissatisfied customers and lost business. On the other hand, a low false positive rate indicates that the system is accurately identifying fraudulent transactions while minimizing disruptions to legitimate transactions.
Calculating and analyzing false positive rates is crucial for balancing fraud detection accuracy, customer satisfaction, and will also reduce the resources required to resolve false positives.
What Causes False Positives?
Numerous factors can contribute to false positives, including incomplete or inaccurate data, technical errors, and more. But the root of the problem essentially boils down to one thing — incorrectly defined rules.
This leads to inaccurate suspicious activity detection, which, when acted upon, turns valid customers away.
Technically, rules can be either too strict or too loose. Typically, the looser they are, the more false positives they’ll pick up, as they’re casting too wide of a net.
So if you have strict rules that aren’t returning a lot of false positives, you have well-defined rules — right? While that’s great in theory, it’s not quite the full picture. What if you just aren’t seeing the fraud at all? What if you’re looking in the wrong place, and the rules you’re currently using are missing your biggest threats?
If rules are set up improperly — and are targeting the wrong behavior and signals — they’ll fail to catch true fraud.
The Impact of False Positives in Fraud Detection
The fact is, false positives hurt an organization's bottom line, costing you lost business — and the revenue that comes with it. Not only that, it has reputational ramifications as well, as customers are receiving inadequate service.
That being said, when left unchecked, fraud losses can pile up significantly, making it an essential task for organizations fighting fraud threats. While false positives come with lost revenue and reputational damage, failing to detect actual fraud can have the same consequences — and often on a much larger scale.
Below, we cover some of the biggest impacts false positives have on organizations:
Valid Customers Get Turned Away
With false positives in fraud, you aren’t actually stopping instances of fraud - you’re actually turning away legitimate customers (and their business). This directly blocks income and gives that user a bad experience with your service.
They may go to a competitor to finish this purchase, and may never return to your platform again. Ultimately, this is the root cause of all the other tangible damages false positives lead to, including a loss in revenue, reputation, and potential future business.
Revenue is Lost
Each instance of a false positive represents a legitimate transaction that was blocked by your fraud prevention system. In essence, you’re actually blocking a legitimate transaction, and turning away perfectly good business.
When added up, this costs organizations substantial amounts in lost revenue.
Reputational Damage
A false positive creates a bad experience for the user. Rightly so, as they are falsely flagged for fraud and subsequently blocked from conducting legitimate business. Whether or not this is rectified, this could give customers a negative impression of your service — or entire brand.
Customers will not only need to go to a competitor for this purchase, but they may shift to a competitor for all future purchases as well. They can leave negative reviews and feedback, which can also damage your reputation.
Wasted Resources and Costs
Every false positive has a cost — there's the value of the blocked transaction itself, the value of investigative resources, and engineering resources that need to be invested into fixing the detection rules.
Every false positive your team investigates puts a direct strain on your risk management team and ties up resources that could have been invested elsewhere. This translates into wasted operational resources, increased operational costs, and a lower return on investment.
How to Reduce False Positives
Reducing false positives is a constant balancing act — you need to catch as much fraud and money laundering as possible without burdening or adding friction to legitimate users. The looser your rules, the more fraud gets through; the tighter your rules, the more false positives you have.
Reducing fraudulent false positives comes down to refining rules to ensure you’re eliminating false positives, without allowing more fraud to pass through undetected. It’s a fine balance, and it’s extremely difficult to perfect.
To effectively reduce false positives, you really need to focus on one thing — refine rules for better accuracy and performance.
The problem is that this is much easier said than done. To actually achieve this, you’ll need to do a few basic steps (the downside — you have to do them over and over again).
- Set an operational baseline: To be able to identify where you need to improve and properly analyze how to make the most impactful changes, you’ll need to have analytics guiding your decision-making process. To start, you’ll need to establish an operational baseline that you can improve on.
- Categorize risk: Some threats are more credible or dangerous than others and therefore pose a greater risk. To conduct effective risk management, it’s important to categorize risk so that cases — and threats — can be prioritized efficiently.
- Refine rules: Rules need to be reevaluated and updated regularly to better detect fraud and keep false positives low. This will require significant engineering resources to update, test, and implement rules that better detect fraud.
Risk management teams are only as effective as the rules they can build for the fraud they’re seeing. To detect and prevent fraud, rules need to be clearly defined and well-thought out so they can actually catch — and empower teams to stop — instances of fraud.
No matter what, this will be a continuous process. Teams will need to create, update, and refine rules consistently for increased accuracy and better performance. Test how new and updated rules perform — if false positives rise, then the rules are catching too much legitimate activity and likely need to be more focused.
Be careful as false positive rates go down, as it doesn’t always mean your rules are performing better. It can just as likely be a signal that fraudsters are getting better at skirting your protective measures. Always revisit your rules, testing and refining them for better detection and fewer false positives.
Check out how we were able to get both Bakkt and Flutterwave’s false positive rates below 15% — and how we were able to help LINE automate false positive resolution by 60% in their first 90 days of using Unit21.
Then schedule a demo to learn how we can help your team reduce false positives and fight off the fraud that matters.