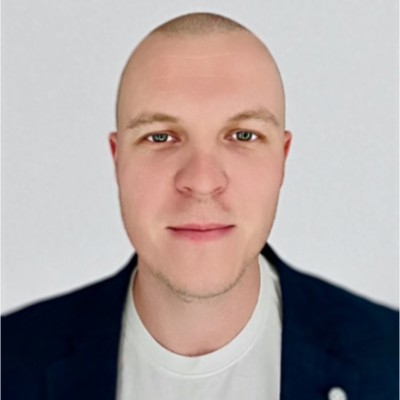
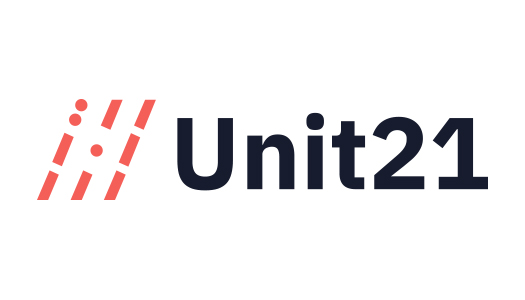
True or false?
1+1=3.
In the case of combining the right matched criteria data points to achieve exponential results and stop more fraud while decreasing false positives- we say true!
Data is Key to Advanced Fraud Detection & Prevention
This is our seventh installment in Fraud Files, our blog series that shares unique insights based on data from over 4.05B events on the Unit21 platform. Our latest posts took a deep dive into the power of data on several trends and insights. Here are a few of our most recent posts, including:
- Part 4: A look into recent fraud trends based on rule creation data
- Part 5: The reasons why precision in data points combined with graph-based rules sharpens fraud detection.
- Part 6: How action-based events can provide real-time indicators of potentially fraudulent or unauthorized activity.
(Read our entire Fraud Files series here.)
The Magic Mix: Combining Match Criteria Increases True Positive Rates
Last month, we explored how impactful precise data points are regarding true positive rates in fraud detection. When accurately collected and verified, the percentages of true positive rates for matched criteria such as phone number (95.21%) and physical address (85.02%) reflect their high reliability as indicators for legitimate fraudulent activity. Combining these precision data points with graph analysis (graph-based rules) makes a powerful tool for fighting fraud.
Using just one match criteria point does not go far enough; in this case, 1+1=1.
Identifying and leveraging a combination of multiple match criteria that are right for your organization’s objectives greatly increases the true positive rate.
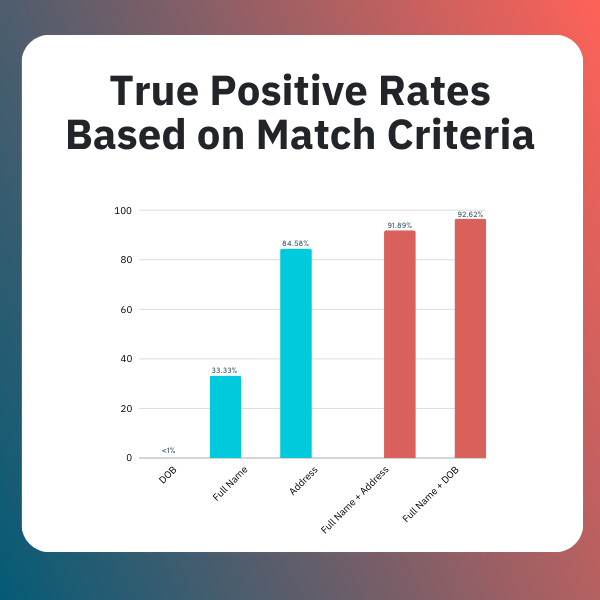
For example, data shows that a full name (33.33%) or date of birth (DOB <1%) as a standalone isn’t very effective, but combining them raises the true positive rates significantly to a whopping 92.62%. Combining phone number and address is also true, where the data reflects a 91.89% true positive rate.
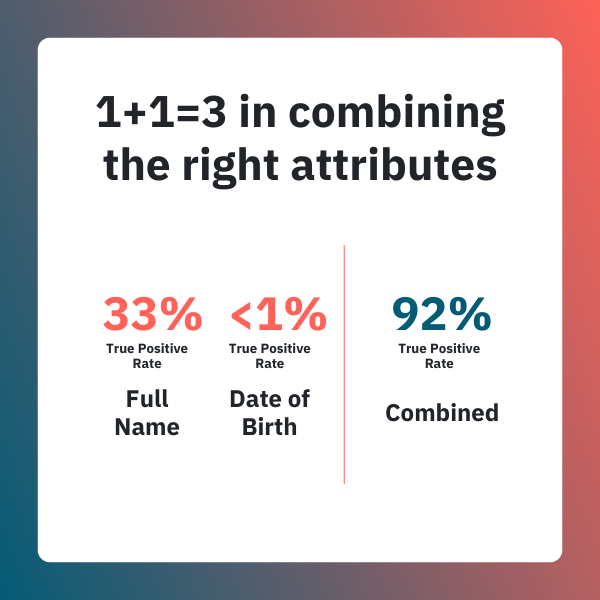
Why is this the case? Our hypothesis is that, in most organizations, there is logic built into the onboarding/KYC flow that blocks users from opening accounts if some elements of the Personal Identifiable Information (PII) already exist for a different user, e.g., the same Social Security Number, phone, and email. While full names and DOBs are not common during these processes, the chances of having two or more users with this match or two people with the same name living at the same address, is rare and/or fraudulent.
False Positive Rates: Just As Crucial As True Positive Rates
The correct combination of precise data points can cause true positive rates to soar, which is important for any risk team. Yet, on the other hand, reducing false positive rates is equally important - and just as rules that combine the correct attributes increase true positive rates, they can also reduce false positive ones.
Many factors can contribute to false positives - technical errors, inaccurate data, etc. - but most can be attributed to one thing: incorrectly defined rules. Too loose of rules, you may be casting too wide of a net, picking up more false positives. Too strict of rules might lead to a lower false positive rate that doesn’t necessarily reflect true fraud. It could mean that your rules are actually missing the biggest threats because you’re looking in the wrong place for them.
At the end of the day, inaccurate fraud detection leads to legitimate transactions being blocked, lost revenue, reputational damage, and wasted resources and costs.
Reducing False Positive Rates
The constant struggle to reduce the rate of false positives without allowing true positives to escape requires strategic planning and optimization to ensure that you are working within a top-performing fraud prevention framework.
Manually reviewing alerts is extremely labor-intensive and resource-hungry. This is where rules-based engines come into play, which can detect suspicious activity and then send that alert to the fraud team to investigate based on the potential threat. These rules need to be continuously evaluated and updated to better detect fraud and lower false positive rates, especially as your organization - and fraudsters themselves - continue to evolve and change. Setting an operational baseline, categorizing risk, and continuously refining the aforementioned rules will go a long way in reducing your false positive rate.
The Takeaway
False positives will never hit zero. But that doesn’t mean you can’t drastically reduce that number with the correct combination of match criteria and comprehensive fraud detection technology that leverages well-thought and clearly defined rules. The goal is to get false positives as low as possible without allowing true instances of fraud to pass through undetected.
Learn more about reducing false positive rates in action: check out how we were able to get global crypto solution Bakkt and African fintech payment infrastructure Flutterwave’s false positive rates below 15% - and how we helped the cryptocurrency arm of LINE, one of the largest messaging apps throughout Asia-Pacific, automate false positive revolution by 60% in their first 90 days of using Unit21.
To discover how Unit21 can help your organization reduce false positive rates, schedule a demo.
Subscribe to our Blog!
Please fill out the form below: