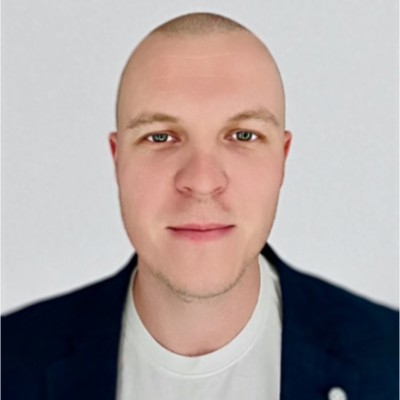
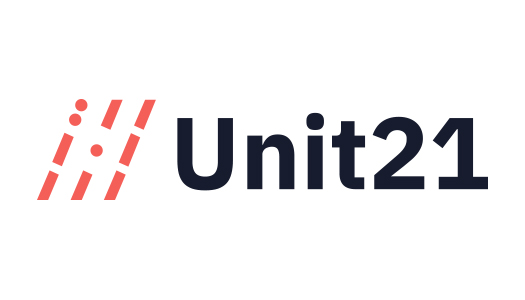
Each industry is unique when it comes to fraud detection and prevention strategies. This rings true, especially when comparing banks and credit unions with fintechs. This is the second part of our two-part installment that dives into the different approaches toward rule types for fraud detection for traditional financial institutions (FIs) and fintechs.
This second part of the installment will focus on fintechs (you can read the first part of the series on traditional FIs here). After reviewing the data, we found that fintechs are taking advantage of our Dynamic Model Builder (DMB) rule engine - a sharp contrast to FIs who often depend on Simple Filters.
Fraud Files: Using the Right Data and Dynamic Rules Are Key to Fighting Fraud
This is our 11th installment in Fraud Files, our blog series that shares unique insights based on data from over 4.05B events on the Unit21 platform. We have been examining how to connect the right data points to detect - and prevent - fraud, why dynamic rules are so important, and what rules traditional banks and credit unions have been leveraging most. Some recent posts include:
- Part 8: How Kevin Bacon can be a good example for linking entities within a network to identify potential fraud rings.
- Part 9: Why Bob Dylan’s song “The Times They Are A-Changin’” is an excellent metaphor for the dynamic nature of fraud detection in a new era of account tenure.
- Part 10: Why banks and credit unions are enamored with Simple Filters - and what is the trade-off for them.
You can read our entire Fraud Files series here.
Top Rule “Types” for Fintechs: A Snapshot
A quick snapshot of the data gives us the following observations:
- Fintechs heavily adopt our Dynamic Model Builder (DMB) rule engine, with almost 60% of their rules written in it
- While we explored how heavily banks and credit unions rely on simple filters, fintechs tend to shy away with less than 3% of their rules running on that scenario.
- Graph-Based Rules (GBRs) are the eighth most common scenario for fintechs - whereas GBRs are not a rule type in the top 10 for banks and credit unions.
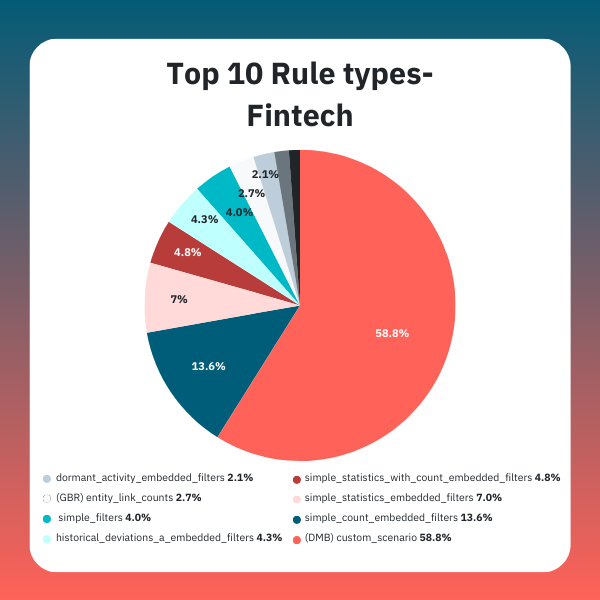
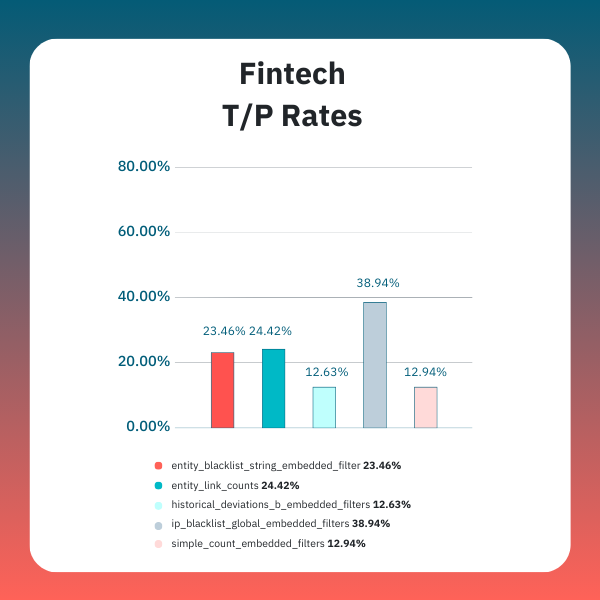
Dynamic Model Builder (DMB) Dominance for Fintechs
As noted before, fintechs often leverage our DMB rule engine, which they use for nearly 60% of their rules. Dynamic Models allow teams to design rules by creating formulas (i.e., alert-generating logic) using variables and trigger conditions, allowing fintechs to be more surgical in designing trigger conditions.
The DMB rule engine allows for flexibility and the ability to tailor fraud detection to complex, fast-evolving transaction patterns, which empower fintechs to stop more fraud. In fact, the flexibility comes from ingesting various types of data, referring to that data when designing variables, and finally using complex formulas to trigger those variables when needed.
The Effectiveness of Graph-Based Rules (GBR) for Fintechs
Graph-based rules - at the eighth most common scenario - help fintechs effectively map out fraudulent networks, spotting sophisticated fraud schemes through entity link analysis. GBR allows teams to visually uncover how entities are interconnected through data. Once suspicious accounts are identified, they can be labeled with tags that allow risk teams to create rules automatically preventing those fraudsters from accessing the platform with different information. GBR can be used in multiple use cases, such as ban evasion and fraud-ring detection.
M1 and Graph-Based Rules: Outperforming Other Rule Types
M1 Finance is a fintech offering a robo-advisory investment platform with various types of accounts and lines of credit, as well as an electronic trading platform, margin lending, and other financial management services.
Their fraud team launched GBRs after typically performing manual investigations on fraudulent users to determine whether they are part of a fraud ring. This was done by manually examining individual pieces of personally identifiable information (PII), such as external bank connections, phone numbers, names, and addresses, for each suspected fraudster. If any other user shared PII with the suspected fraudster, they were flagged as suspected or confirmed fraud, based on the uniqueness of the identifying information. This investigation usually took place after the user had already made transactions.
M1’s fraud team created Duplicity Rules (looking for duplicate data among users) with the goal of detecting users with matching PII at the onset of their interaction with the M1 platform since this activity is usually indicative of suspicious behavior. Users are typically identified at the beginning of the funnel before they can transact. For example, as soon as a bank account is added when a user first creates an M1 account, the rule will flag a suspicious user typically before they transact using that account.
Since the launch of these rules, M1 has consistently exceeded expectations, with an average efficacy rate of 52.08% for Matching Bank Account and 55.18% for Matching Phone Number. More than 58 net new users have been identified for sharing PII with a network of other users on the platform, resulting in a “Confirmed Fraud” status.
High Performance with a Tech Focus
The overall performance metrics for fintechs at 14.06% true positive rate are more efficient than banks and credit unions at 8.09% true positive rate. Fintechs, by nature, tend to be more tech-driven and are able to be flexible and adaptable at scale much easier than legacy institutions. A good example of this is seen in the most effective scenario for fintechs, IP Blacklist (Global), with close to 39% T/P rate. This triggers an alert when a user or institution engages in a transaction from a known blacklisted IP address. Because traditional FIs see many transactions with no IP data - e.g., branch check deposits, ATM transactions, and card swipes - they are not able to leverage IP-based scenarios as effectively. It is more common for fintechs simply because their operation/transactions rely heavily on online activity, and IP address information can be mined.
Historical deviation filters - one of our recommended general rules - also show substantial effectiveness in fintech environments with a combined 17.08% T/P rate. The two types of historical deviation filters - A and B - can look for anomalous behaviors. For example, teams can generate an alert if the transaction amount falls outside of the expected distribution (Historical Deviations A) and/or generate an alert if the transaction total amount differs by a specific amount (Historical Deviations B).
The Takeaway
We see how the fintech sector - by its own nature - allows it to leverage flexibility, adaptability, and technology, which, in turn, helps it succeed in its fraud detection and prevention efforts. While there is, of course, efficacy in the more static, traditional approaches, the fintech approach of integrating more complex rule types - implemented in a measured and risk-aware manner - combined with dynamic rules makes up a powerful fraud prevention strategy. By nature, a fintech company might have fewer manpower resources, so they focus on being more efficient as they have fewer people to work the alerts.
If you are looking for some practical tips on writing dynamic rules, join our Rule Building Master Class during our Fraud Fighters Virtual Summit. The summit takes place on July 17, and the Rules Building Masterclass is just one of more than 20 practical sessions that can take your fraud-fighting skills to the next level.
Subscribe to our Blog!
Please fill out the form below: